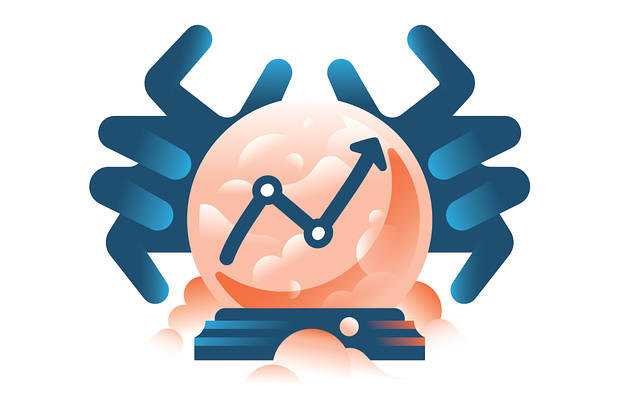
Prediction with Mixed Effects Models: A Monte Carlo Simulation Study
Anthony Mangino
Ball State University
Data within the social sciences are frequently nested in structure such that individuals are members of higher-level groups. Consequently, this requires use of multilevel models accounting for this structure. Within two-group classification tasks, the most common method, the generalized linear mixed effects model (GLMML), may not provide ideal classification accuracy. This study uses a Monte Carlo simulation to compare a number of multilevel classification methods, including GLMML, random effects expectation minimization trees (REEMTree), mixed effects random forests (MERF), mixed effects gradient boosting machines (Megbm), panel neural networks (PNNET), and a Bayesian framework (Bayes), on their classification accuracy under a number of data conditions. The conditions differed across group size ratio, group separation, sample size at level-1 and level-2, and within- and between-group correlations. Results indicate that performance broadly differs across methods. Broadly, Bayes and PNNET tended to outperform all other methods in varied conditions, including those in which within-group correlations became increasingly larger. All other methods tended to perform comparably to one another under the other conditions presently tested. The present study provides researchers on insights into which of these methods perform optimally under varying conditions to improve statistical practice with multilevel data.
Note: Completed manuscript currently in first-round revisions with Educational and Psychological Measurement. Full in-progress citation is Mangino, A.A. & Finch, W.H. (2020). Prediction with mixed effects models: A Monte Carlo simulation study [Manuscript submitted for publication]. Department of Educational Psychology, Ball State University.
Anthony A. Mangino, M.S. – Tony Mangino is a third-year doctoral student in the Department of Educational Psychology at Ball State University. His primary research interests are in areas of classification analyses, multilevel modeling, research design and consultation, text analysis, and simulation methodology. Tony is currently a consultant at the Research Design Studio under the direction of Drs. Jerrell Cassady and Matthew Stuve. His dissertation committee chair is Dr. Jocelyn Bolin, who has served as his mentor since beginning the doctoral program.